When data is sparse...
- Brandon Sodenkamp
- Jan 24, 2019
- 2 min read
An unfortunate truth of data science is that even when the data read zero, your processor must account for it. Now, if a majority of the data is zero, it can often feel as though you are using a tow truck to haul a bike; that is just overkill.
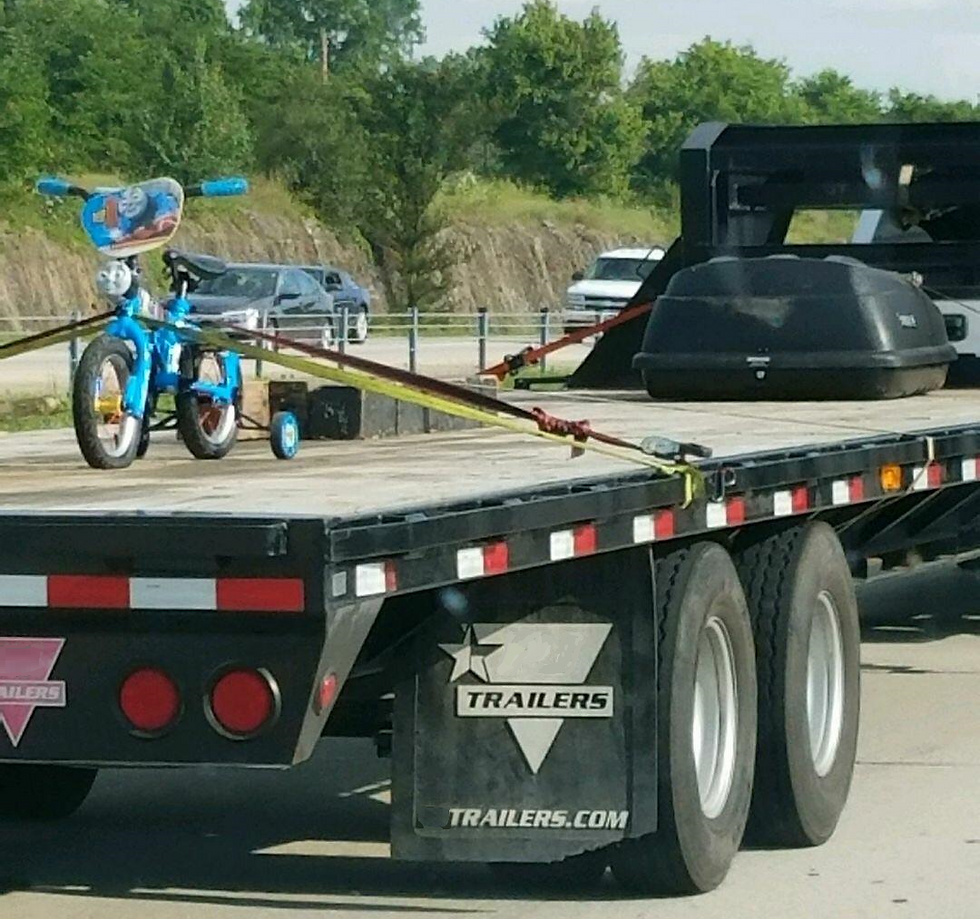
To address this, a data scientist from Anaconda named Matt Rocklin began working on a solution in his spare time. Eventually, the concept grew into a full project, and it was at this point when Hameer Abbasi hopped on board because of a project involving tensor factorization. Hameer is a scientific software developer at Quansight and lead developer of PyData/Sparse. Explaining how he got involved, Hameer, during our Open Source Directions webinar said, “there was an easy way to do multidimensional sparse arrays in Matlab, but not in Python, as a result, I decided to join this project as it seemed most promising.” In addition to the work by Hameer and Matt, Ralf Gommers has also contributed ideas and direction to the project development. Though some other contributors have assisted in putting this project together, Hameer remains the main consistent presence in the project.
PyData/Sparse is a project that aims to provide ndarray-compatible containers for storing sparse arrays, and implementing NumPy methods that act on those containers. At the moment this functionality is somewhat unique in the PyData ecosystem because there are not many sparse containers available. Recently PyData/Sparse also underwent its first major library integration with TensorLy. Aside from TensorLy users, academia has taken to this project most readily and it is currently being utilized at universities.
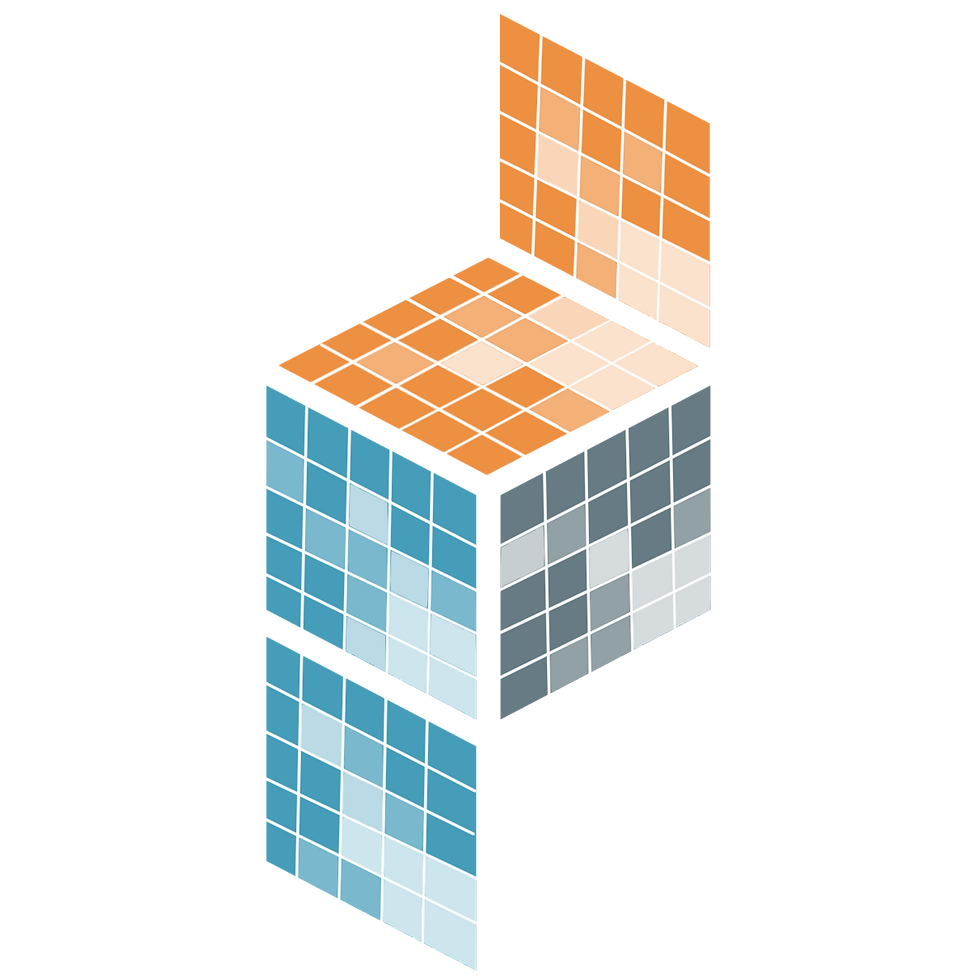
One of the most important developments that will come along with PyData/Sparse is a new compression format. Currently, SciPy has a format called compressed sparse column and compressed sparse row format. The good thing about both of these formats is that they take up less space than COO in most situations. The new file format would be called compressed sparse fibers or CSF and would be more than just a memory characterization. Creating this new format would allow for zero overhead when passing into these libraries or getting data out of these libraries. Ultimately it would become more of a catalyst to adding future functionalities and getting rid of scipy.sparse.
This project is still fairly new and holds a great deal of potential. As such it will need more assistance if it is to reach the goals that have been set. Among the objectives are
Sorting coordinates to be C contiguous just as they would in NumPy
Providing BLAS support
Get PyData/Sparse to work with SciPy’s most recent linear algebra routines
Dask integration for high scalability
Make sparse arrays faster possibly utilizing things like the Cuda routines with CuPy
Test infrastructure overhaul
For more information about the project, and what it seeks to accomplish, see the website here, and the roadmap here.
Comments