Parallel processing, unparalleled flexibility
- Brandon Sodenkamp
- Feb 7, 2019
- 3 min read
Managing the processing power, whether for individual CPU cores or entire computer grids, is essential when you are trying to get the most out of your data crunching. Dask continues to be an essential tool in the Python data science ecosystem to achieve the goal of cutting down processing time by maximizing parallelization. The number of users has grown rapidly since its initial release, and today there are about 250,000 downloads per month on Conda-forge and an additional 1 million downloads on PyPI. In this way, Dask has become an established part of the PyData ecosystem.
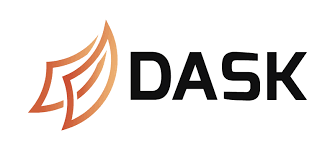
For the episode of Open Source Directions featuring this project, Jim Crist and Tom Augspurger explained more about the project and what the thoughts were about its potential going forward. Jim Crist is a software developer at Anaconda and works on Dask in addition to other projects. Tom Augspurger, is also a software developer at Anaconda and works on Dask as well as open-source packages like Pandas.
Dask began around the end of 2014/beginning of 2015 when it spun out of the Blaze library and ecosystem project. Matt Rocklin was the original author of Dask, and as the story goes, one Christmas break he began to toy around with a new idea which he brought back to the office. This idea would later be used to scale up Python computing as just a back end component. It was later realized that Dask could be quite a useful tool on its own, but at this time most libraries in the scientific Python world were single threaded (meaning they only take one core) and were limited to in-memory datasets so you couldn't do large computations. Dask became a way to take the existing ecosystem and scale it up to solve larger problems by using all the cores or all the machines on a cluster without having to rewrite the ecosystem. After the use case was established for Dask, they decided that this project would also emphasize making an interface that is familiar to users. These innovations and efforts are likely the reason that this project has become so ubiquitous today.
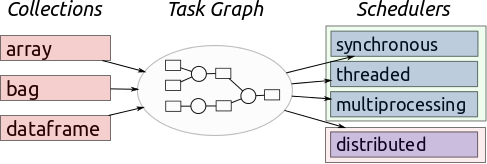
Because Dask has become a sort of ecosystem of its own, there are now many maintainers with various areas of expertise and it remains at the forefront of data science development. Frequently there will be individuals who only focus on maintaining one element of the overall project because of the ecosystem model, which has helped to ensure consistent progress among its various components.
Looking ahead at the future of this project, there are a few wishlist items that are currently being looked at but at the same time, the desire is to keep this an open source project which is able to be shaped by the community according to the needs of the community. Establishing formal governance is first up on the list, and you can find the started document in the GitHub repository here. The benefits of establishing a governance model have become more apparent as the project and team have grown, especially when talking about working with external groups. In addition to governance, Gradient boosted trees are another exciting development on the horizon. Currently, the user would just use a Dask XGBoost package, but Olivier Brosseau and an associate of his are working on a pure Python and Numba accelerated gradient boosted library. These are just a couple of wishlist items, and anyone is welcome to add to this list which can be found here. As this project continues to evolve it will be interesting to see what new developments come through the direction of the ever-growing community.
Comentarios